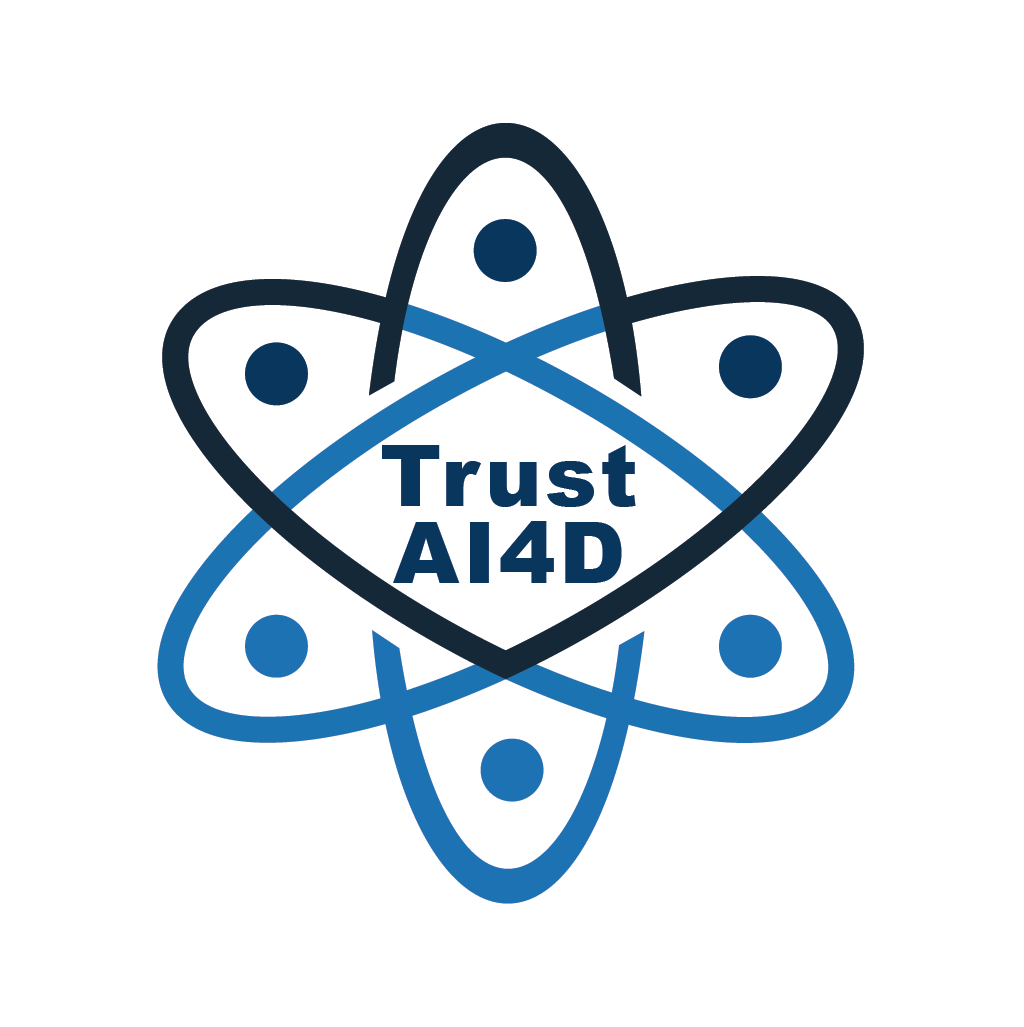
TRUST-AI4D
AI methods have unique potential to produce clinically valuable insights from large-scale, heterogeneous patient data. However, existing approaches using multifaceted data to predict treatment response and disease state are often rather blunt tools without the ability to query and interpret in a meaningful way why a given prediction or decision has been suggested.
In order to effectively manage diabetic patients and forecast the likelihood of complications, the trustworthiness of AI algorithms hinges upon the interpretability of their outcomes. This quality of reliability is crucial to ensure their applicability in clinical practice, as it promotes comprehension and acceptance among doctors and patients.
The TRUST-AI4D project will simultaneously improve the interpretability and trustworthiness of AI approaches using large-scale patient data to predict diabetes disease progression and complications – with substantial potential for generalization to other AI applications. The goal is to create easy-to-use artificial intelligence (AI) tools for medical doctors and patients to enable early detection of deterioration in organs’ function that often suffer in patients with diabetes such as the kidneys, eyes, nerves and heart. This will be a critical breakthrough in ‘trustworthy AI’, and utilization of AI tools for clinical decision support and deciphering disease mechanisms.
The TRUST-AI4D project will improve available AI algorithms for prediction of treatment response and diabetes complications by making them more fair, explainable and trustworthy – with high generalizability to other applications.
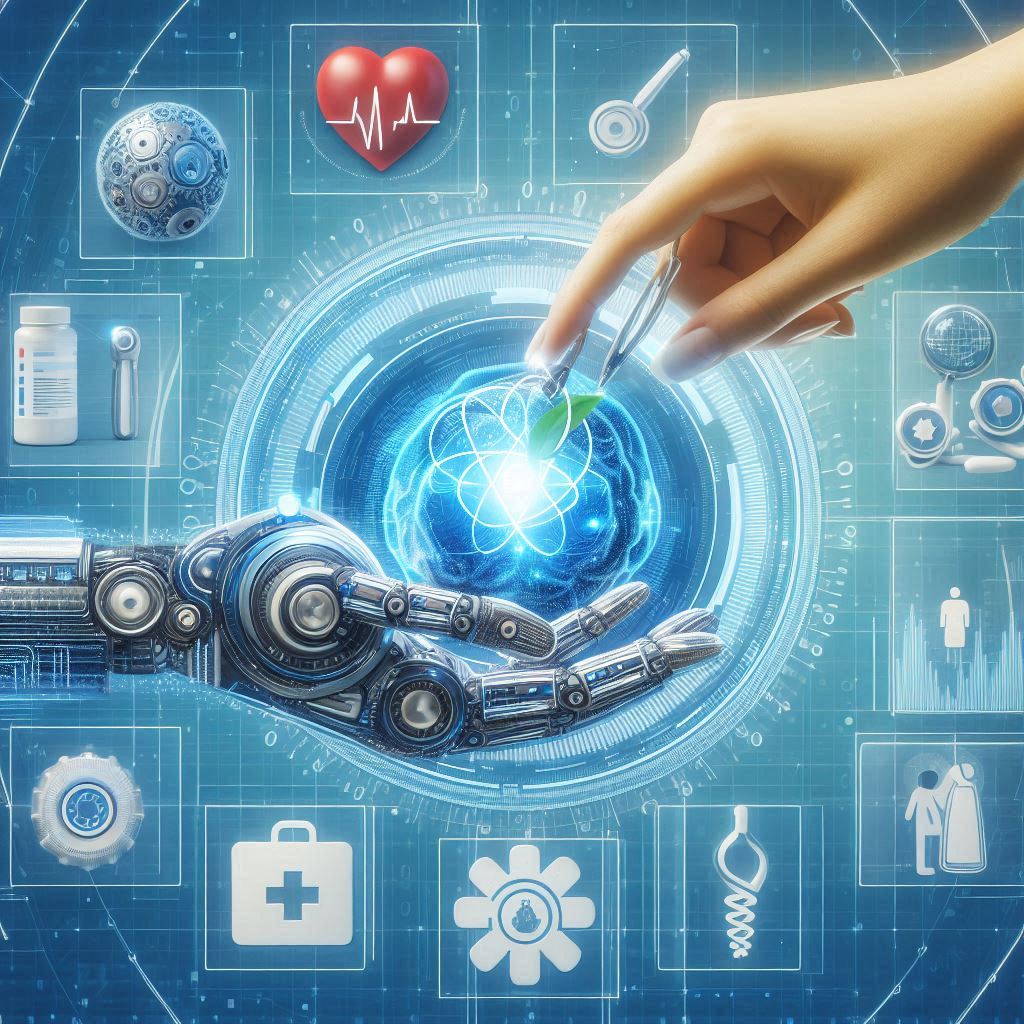
AI generated using Microsoft Designer
Primary Objectives
TRUST-AI4D will simultaneously improve interpretability and trustworthiness of AI approaches using large-scale patient data to predict diabetes disease progression and complications. It will achieve this by coupling interpretable machine learning methods for classification and dimensionality reduction into a newly established and powerful “hypercubic inference” modelling framework for predicting disease dynamics. This approach will retain the ability to trace back every prediction about future behaviour with and without interventions to the learned parameters. The provenance of every prediction will therefore be immediately examinable.
Secondary Objectives
1. To develop an interpretable AI-based environment for selecting and cataloguing diabetes and complications related multiomic features (WP1).
2. To couple the outputs of WP1 to predict diabetes complications by implementation of trustworthy and explainable hypercubic inference algorithms (WP2).
3. To validate this coupled, interpretable algorithm and investigate trustworthiness, fairness and ethics of the algorithm and its outcomes in the real-world setting (WP3).
4. To foster and facilitate dissemination of the AI methods into biomedical research and best clinical practice of personalised medicine (WP4).
More about the Trust-AI4D project here: